Research
Subsurface uncertainty quantification
Project overview
The sustainability of coastal river-deltas is impacted by a multitude of natural and anthropogenic factors, including a limited understanding of subsurface flow patterns. This project uses information theoretic metrics and machine learning models to constrain variability in the subsurface, and therefore contributes to societal sustainability on river-deltas.
This research is funded by an NSF Postdoctoral Fellowship from 2020–2022.
Introduction
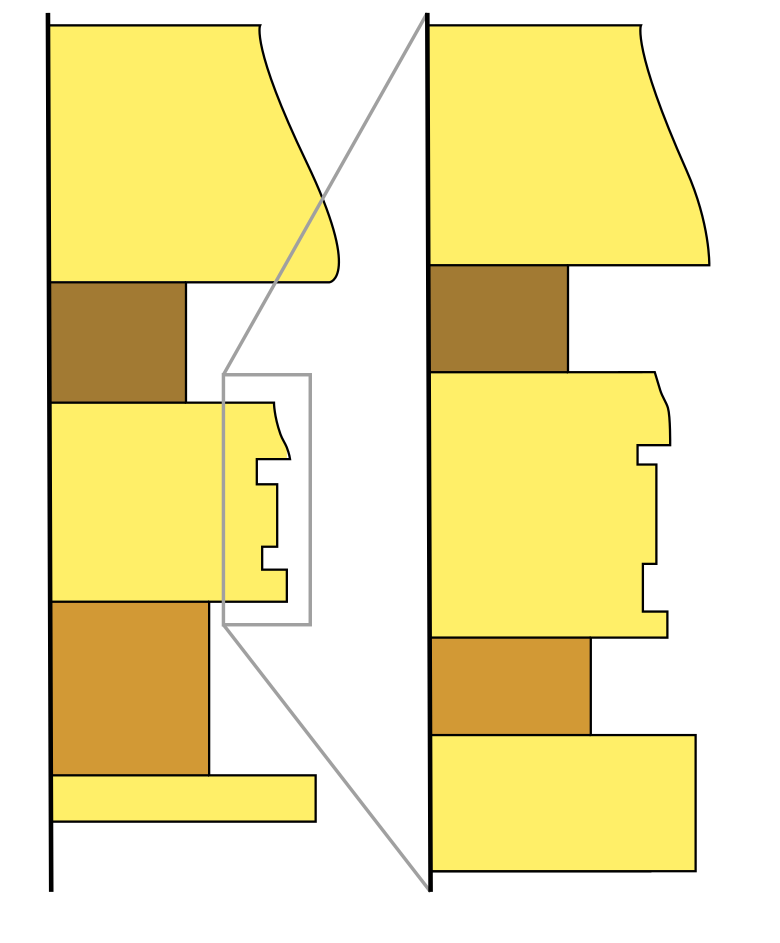
Geological heterogeneity strongly influences flow pathways and thus rates of contaminant transport and groundwater aquifer recharge, which limits our ability to sustainably manage water resources and mitigate health risks in river-delta environments. Spatial scales of subsurface stratigraphic variability in river-deltas ranges from micron-scale pore-space discontinuities to irregularly-shaped sand-bodies that can be tens of meters thick and many kilometers in length. Smaller-scale subsurface heterogeneity due to channel and bedform dynamics (<1~m) is typically under-constrained, because it is below the resolution that can be imaged by geophysical techniques.
Theory and some evidence suggests that stratigraphic sequences may be scale invariant (Figure 1), which opens a pathway to constrain smaller-scale heterogeneity via observation of larger-scale heterogeneity. This project directly addresses the question: can patterns and information gleaned from subsurface heterogeneity at one spatial scale be used to constrain uncertainty at another scale?
Methods
One model produced by this project is a deep-convolutional generative adversarial network (StratGAN) that has learned to produce synthetic synthetic fluvial stratigraphy, where black pixels represent channel, and white is non-channel. A novel contribution of this research project was coupling texture-synthesis algorithms (Hoffimann et al., 2017) with conditional in-painting optimization (Dupont et al., 2018), so to “scale up” realizations from the machine learning model, while honoring ground-truth data (e.g., cores).
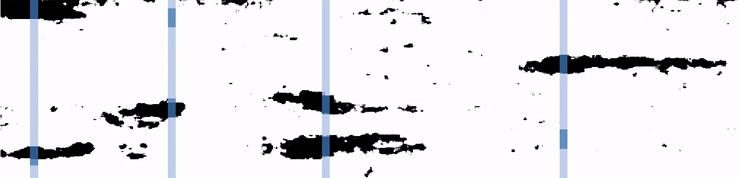
Additionally, measures from information theory are used to quantify scale invariance in stratigraphy. For example, a break in scale invariance is identified by step-wise reduction in mutual information within a vertical stratigraphic sequence.
Insights
StratGAN reduces subsurface uncertainty through a large sampling of realistic potential rock geometries throughout a reservoir, without any a priori assumptions about the geology. The model is a proof-of-concept for using GANs in subsurface uncertainty quantification. Current work is developing methods which enable insertion of geological domain expertise into synthetic realizations of generative models via representation latent encoding.
Publications generated by this research
There are no peer reviewed publications from this work yet, but you can learn more about the StratGAN model on GitHub.
References
Hoffimann, J., Scheidt, C., Barfod, A., & Caers, J. (2017). Stochastic simulation by image quilting of process-based geological models. Computers & Geosciences, 106, 18–32. doi: 10.1016/j.cageo.2017.05.012
Dupont, E., Zhang, T., Tilke, P., Liang, L., & Bailey, W. (2018). Generating Realistic Geology Conditioned on Physical Measurements with Generative Adversarial Networks. ArXiv [ML]. [link]